Timely and accurate digital data that is accessible for insight is the goal of many organizations and U.S. federal agencies. However, the data landscape has significantly changed in the last decade and many organizations have not adequately updated their data management and monitoring with proactive data governance practices and tools.
The White House released a new National Cybersecurity Strategy in March 2023 that makes it clear that organizations will be held responsible for data protection – it’s a national priority and a responsibility to U.S. citizens.
Data Governance Is a Social & Legal Responsibility
Technology is moving fast and data is growing fast – organizations and federal guidance have struggled to keep pace. People have difficulty changing their minds and habits, and change is therefore even harder for organizations made up of many people, legacy tools and equipment, organizational habits, and established processes.
Yet change is an imperative and organizations that wish to harvest their data for insights must also be responsible for revamping data governance practices to protect individuals and other organizations from harm due to a lack of data controls.
Cybercriminals and countries like China and Russia continue to take advantage of organizational inertia towards data protection. In addition to these risks, artificial intelligence is comprised of algorithms that follow steps based on data inputs, and without controlling for data quality and bias, harmful decision-making can result.
Three data governance areas of concern include:
- Comprehensive Data Discovery & Classification – Data identification, classification, and monitoring for cybersecurity controls, retention policies, quality data analytics, and reduction of storage costs.
- Data Risk Management During Digital Transformation – Data quality control, sensitive data management, and policy assignment during digitization and digital transformation projects (physical and digital records).
- Sensitive Data Identification & Management for Analytics – Data privacy and sensitive data identification, mapping, and risk management. Implementing redaction and anonymization for data analytics projects and information requests.
These three challenges, if not properly managed, create significant data security and compliance issues that result in questionable data quality for decision-making, create privacy regulation risks, and contribute to excessive IT resource and storage costs. Often data is unable to be incorporated into projects in a timely manner because it can’t be properly identified and controlled.
A recent TechTarget article makes a good point with a quote from Matt McGivern, a managing director at Protiviti, a management consulting firm:
“It has become popular to call data an asset, but without proper data governance, this is impossible.”
More regulation is likely coming with the March 2, 2023 release of the much-awaited White House National Cybersecurity Strategy, which takes a new strong stance against risks that have accompanied our shift towards a digital world, accelerated by the COVID-19 pandemic. The strategy warns that, “Digital connectivity should be a tool that uplifts and empowers people everywhere, not one used for repression or coercion.”
Haphazard data governance is no longer acceptable in a world with data growing exponentially, Internet of Things (IoT) devices and other connectivity risks, and emerging technology risks from artificial intelligence and quantum computing.
Cyberthreats have increased in sophistication, frequently targeting poor cyber hygiene and human habits. Organizations will need to use risk management to identify sensitive data, users and devices who access the data, and the people, organizational habits, and environment that put data at risk.
Proactive prevention of risk during digitization and digital transformation projects should be the goal rather than waiting until a problem arises later that can be costly to fix and leads to fines and reputation damage.
Five pillars support the national cybersecurity strategy, including:
- Defend Critical Infrastructure
- Target and Disrupt Threat Actors
- Use Market Forces to Improve Security and Resilience
- Invest in Resilience
- Enhance International Partnerships
The third pillar, “Use Market Forces to Improve Security and Resilience,” focuses on “promoting the privacy and security of personal data” and seeks to drive data holders to better secure it.
Key points from that pillar include:
“Continued disruptions of critical infrastructure and thefts of personal data make clear that market forces alone have not been enough to drive broad adoption of best practices in cybersecurity and resilience….We must hold the stewards of our data accountable for the protection of personal data … and reshape the laws that govern liability for data losses and harm caused by cybersecurity errors, software vulnerabilities, and other risks created by software and digital technologies.”
“Securing personal data is a foundational aspect to protecting consumer privacy in a digital future. Data-driven technologies have transformed our economy and offer convenience for consumers. But the dramatic proliferation of personal information expands the threat environment and increases the impact of data breaches on consumers. When organizations that have data on individuals fail to act as responsible stewards for this data, they externalize the costs onto everyday Americans. Often, the greatest harm falls upon the vulnerable populations for whom risks to their personal data can produce disproportionate harms.”
The elderly and children are often targets. As we referenced in our article, “Healthcare Data Privacy, Security, and Accuracy Are Dependent on Safe and Successful Digital Transformation,” hackers are targeting children’s hospitals to use data from pediatric health records to apply for loans. Damage to the patients’ credit may go undetected until victims are adults.
When it comes to healthcare data, electronic personal health information (ePHI) requires special protections. A recent report from healthcare analytics company Protenus Breach Barometer found that 59.7M patient records were breached in 2022, an increase of 18% compared to 2021. And 12% of healthcare data breaches were compromised by insider wrongdoing.
The U.S. government is committed to taking a leadership role in best practices around these cybersecurity strategy pillars, including data protection. Many states have also enacted legislation related to protecting personal data. There are special risks around the unintentional sharing or exposure of healthcare ePHI data, and new legislation and standards continue to be released.
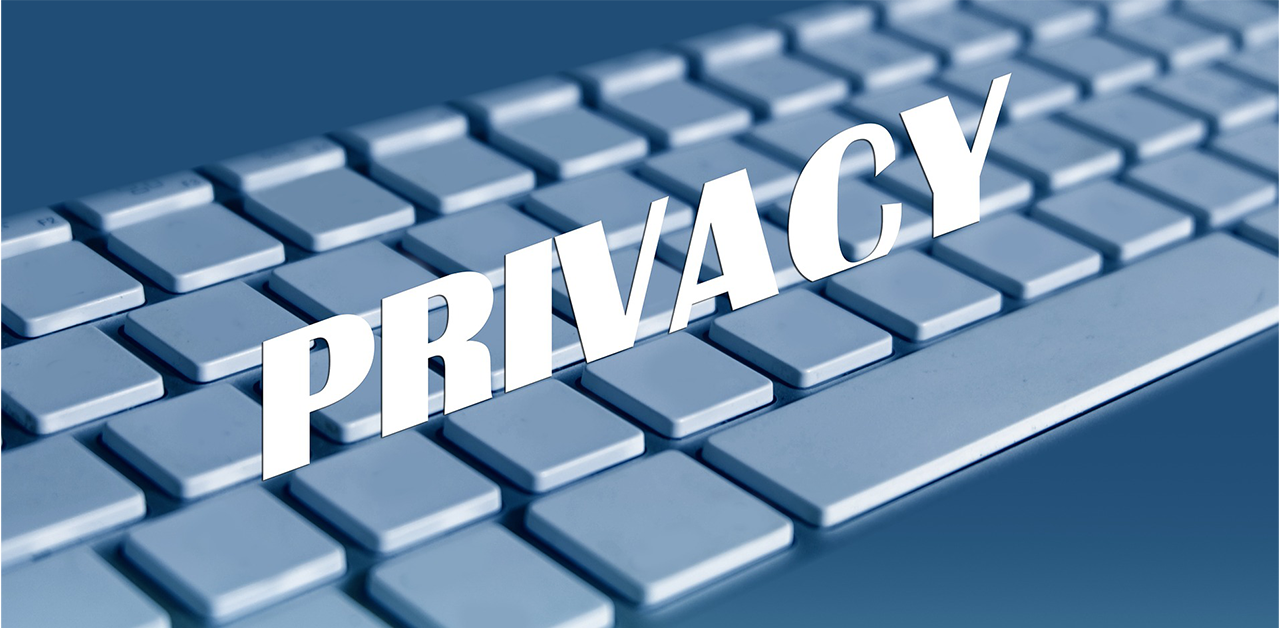
One job of the government is to help nudge (or push!) people and organizations to do what’s socially responsible through regulation. Below are some of the laws U.S. companies, federal agencies, and healthcare organizations need to consider that support data protection:
- Privacy Act of 1974 – Protects records about individuals retrieved by personal identifiers such as a name, social security number, or other identifying number or symbol. An individual has rights under the Privacy Act to seek access to and request correction or an accounting of disclosures of any such records maintained about him or her. Requires written consent by the individuals to disclose their records.
- Freedom of Information Act (FOIA) (1967) – Applies to federal agencies and provides that any person has the right to request access to federal agency records or information except to the extent the records are protected from disclosure by any of nine exemptions contained in the law or by one of three special law enforcement record exclusions.
- Health Insurance Portability and Accountability Act of 1996 – Applies to healthcare covered entities and established national standards to properly protect protected health information (PHI) while allowing the flow of health information needed to provide and promote high-quality health care and to protect the public’s health and well-being.
- The Health Information Technology for Economic and Clinical Health (HITECH) Act 2009 – The HITECH Act has several healthcare objectives, including incentivizing healthcare organizations to move to electronic healthcare records to improve accessibility for patient decision-making.
- 21st Century Cures Act (2016) – The 21st Century Cures Act promotes innovation in the healthcare technology ecosystem to deliver better information, more conveniently, to patients and clinicians. It also promotes transparency by using technology to enable the public to gain visibility in the services, quality, and costs of health care. The final rule includes a provision requiring that patients can electronically access all of their electronic health information, structured and/or unstructured, at no cost.
- General Data Protection Regulation (GDPR) – For U.S. organizations doing business in the European Union (EU), GDPR imposes strict regulations on data privacy and protection for business transactions in their EU member states.
CSO has a comprehensive list of legislation, and below are some of the recent state legislation:
Unfortunately, there are many data quality and security issues that result from poor internal data governance including:
- insider risk from employees removing or tampering data
- human error such as misfiled patient records
- oversharing of files without clear policies
- unprotected sensitive data
- duplicate data without clear data lineage and provenance (where data originated and how it has been changed over time)
- unencrypted credential files or intellectual property
- ROT (Redundant, Obsolete, and Trivial) data causing excessive storage costs and IT resource drain
The National Institute of Standards and Technology (NIST) works to develop best practices and guidance, and recent publications have an increased focus on governance and risk management.
Data Governance Is Critical to Risk Management
Risk management is a people-intensive and leadership-led process of authentically reviewing threats and setting risk appetite and tolerance for an organization. A few of NIST’s recent guidance projects that pertain to data governance include:
- NIST AI Risk Management Framework 1.0 (January 2023) – The AI Risk Management guide discusses that “without proper controls, AI systems can amplify, perpetuate, or exacerbate inequitable or undesirable outcomes for individuals and communities … Core concepts in responsible AI emphasize human centricity, social responsibility, and sustainability. AI risk management can drive responsible uses and practices by prompting organizations and their internal teams who design, develop, and deploy AI to think more critically about context and potential or unexpected negative and positive impacts.”
- NIST Data Classification Project (currently in Build Phase) – NIST outlines the importance of data classification, saying “A critical factor for achieving success in any business is the ability to share information and collaborate effectively and efficiently while satisfying the security and privacy requirements for protecting that information … As part of a Zero Trust approach, data-centric security management aims to enhance protection of information (data) regardless of where the data resides or who it is shared with. Data-centric security management necessarily depends on organizations knowing what data they have, what its characteristics are, and what security and privacy requirements it needs to meet so the necessary protections can be achieved.”
- NIST Cybersecurity Framework 2.0 Concept Paper (January 19, 2023) – After industry input, the updated Cybersecurity Framework 2.0 will likely include a new “Govern” Function to emphasize cybersecurity risk management governance outcomes. This will be added as a crosscutting Function to the existing Functions: Identify, Detect, Protect, Respond, and Recover. NIST states “The new Govern Function in CSF 2.0 will inform and support the other Functions” and a “Govern Function is also consistent with the Govern Functions in the draft AI Risk Management Framework and the Privacy Framework.”
- NIST SP 800-66 Rev. 2 Implementing the Health Insurance Portability and Accountability Act (HIPAA) Security Rule: A Cybersecurity Resource Guide (Draft issued July 21, 2022) – This new HIPAA Security Rule guide heavily emphasizes a risk management approach to protecting ePHI. Risk management begins with requiring that the regulated entity “understand where ePHI is created, received, maintained, processed, or transmitted. Identify where ePHI is generated within the organization, where and how it enters the organization (e.g., web portals), where it moves and flows within the organization (e.g., to specific information systems), where it is stored, and where ePHI leaves the organization.”
Below are a couple of our recent articles that highlight the data asset identification requirements laid out in NISTIR 8286 guide, Integrating Cybersecurity and Enterprise Risk Management (ERM):
The NIST AI Risk Management framework calls out the need for social responsibility and proactive harm prevention rather than minimal compliance. Social responsibility requires leadership-led data governance.
The idea that social responsibility may require stronger legislation is due to the fact that human beings struggle with changing their beliefs, habits, bias, and conditioning even if it is in their own best interest, or in the best interest of their nation or world. Prioritizing competing demands against the status quo is often no easy task.
A few of our articles going over the psychological challenges of creating organizational change for data protection include:
A recent McKinsey & Company interview with Julie Houston, Equifax’s Chief Strategy & Marketing Officer, highlighted how essential culture, leadership, and change management was to their transformation after the massive 2017 Equifax data breach. Houston said Equifax’s CISO often reiterates that “Culture is the difference between good security and great security.”
Now, or in the near future, leadership at organizations must commit their organizations to finding technology solutions and implementing training that will create the cultural changes necessary to tackle data governance.
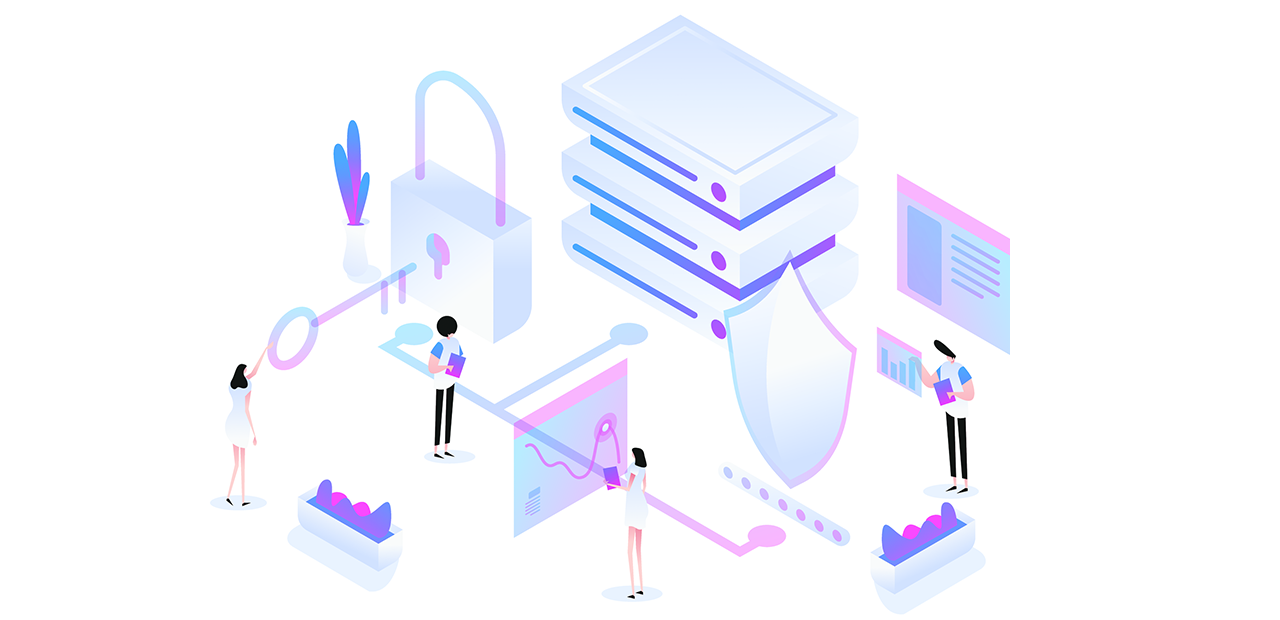
Zero Trust Requires Data Classification & Governance
Data governance is also foundational to Zero Trust. John Kindervag, who helped define Zero Trust at Forrester Research, wrote the 2010 paper “No More Chewy Centers: The Zero-Trust Model Of Information,” and contributed to the President’s National Security Telecommunications Advisory Committee (NSTAC) Draft on Zero Trust and Trusted Identity Management.
In a two-part VentureBeat article, Kindervag was interviewed on Zero Trust and explains how defining Zero Trust must start with defining protect surfaces he refers to as DAAS (Data Assets Applications Services), rather than jumping into buying technology. His five-step model leads with identify your sensitive data and map sensitive data flows.
In part two of the interview, Kindervag also describes the business benefits of Zero Trust, saying:
“The biggest and best-unintended consequence of Zero Trust was how much it improves the ability to deal with compliance, auditors, and things like that.”
He outlined an example of how Zero Trust saved an organization he worked with from any costs for compliance after an audit.
It’s never too late to start making effective data governance a business priority, and with increasing data privacy and cybersecurity regulation, organizations should realize this is becoming a necessity.
Data protection should also be baked into digitization and digital transformation projects with clear audit and control measures, especially when sensitive data is involved.
For organizations that did not implement data classification and policies at the beginning of a project, there are AI/ML data discovery, classification, and intelligent document processing tools that can help quickly automate data indexing, filtering, and flagging across the entire data estate of unstructured and structured data to reduce the manual burden on IT staff.
A couple of our recent articles on Zero Trust data-centric architecture include:
Data Governance Solutions for Data-Based Decisions
Artificial intelligence, machine learning, and natural language processing are increasingly being used for data-based decision-making. But they are only as good as the data. If you have error-prone data, biased data, incomplete data, or misfiled data, decisions can be compromised.
Data is growing at exponential rates from a myriad of devices. Organizations will be better served by proactively managing data now rather than waiting for new legislation that requires data cleansing and protection efforts later on.
Implementing data governance with classification and policies will also improve usability and access to information because controls will be in place to protect sensitive data. Sensitive data often requires redaction and anonymization when used for analytics and research projects, or for information requests.
Analyzing data while preserving privacy is a focus for organizations like the leadership at the Department of Health and Human Services (HHS), especially following the COVID-19 pandemic.
According to a FEDSCOOP article, at a January 2023 AFCEA Bethesda Health IT Summit, HHS Chief Data Officer Nikolaos Ipiotis stated that potentially lifesaving insights often remain untapped because of the length of time it takes staff and agencies to become comfortable with sharing certain assets. Ipiotis said:
“When somebody is asked to share data with someone else, the first thing they are thinking is ‘this is a very daunting process.’ It typically takes months. In fact, when you start drafting a data-sharing agreement with another agency, I would say that more than half die in the process. Because by the time the agreement is signed, the need is not there anymore.”
To prevent future data problems, new bulk data (physical or digital) being ingested into the digital ecosystem should be securely processed and indexed from the beginning with chain-of-custody tracking, audit, sensitive data classification, and workflow controls. This process now is much more efficient when aided by modern, semi-automated Intelligent Document Processing (IDP) solutions.
Data already living in the system can be inventoried, risk assessed, classified, and tagged for user/device controls using AI/ML Data Discovery tools. Inventory and risk management actions are core tenants of Zero Trust microsegmentation.
Automated data discovery and tagging can locate and fix data retention issues or past file-handling mistakes to avoid compliance issues and data quality problems. These solutions also can automate the anonymization and redaction of data so that data can be accessible but protected for research and information requests.
Anacomp’s D3 AI/ML Data Discovery and Intelligent Document Processing (IDP) solutions reduce digital transformation, security, and storage costs by helping you control your data with confidence using automation implemented by our expert professional services staff:
D3 Digital Transformation Solutions
Our Intelligent Document Processing (IDP) and high-speed scanning solutions use technologies like Artificial Intelligence (AI), Machine Learning (ML), Natural Language Processing (NLP), and Optical Character Recognition (OCR) to process and ingest many types of data including handwriting and poor-quality documents, as well as images, enabling you to incorporate more data into records and data projects.
- Quickly, securely, and accurately digitize and classify records
- Flag and correct data-handling mistakes and sensitive data errors to protect record accuracy and prevent fines (such as misfiled records or other HIPAA violations)
- Anonymize or redact data for research and other information requests
- Automate classification and data extraction with minimal operator assistance
We offer secure digitization and indexing of all types of sensitive records for Electronic Health Record (EHR) systems, claims processing, benefits delivery, compliance, data analytics, intellectual property, human capital management, and secure records management.
D3 Data Discovery and Distillation Solution
Our Data Discovery and Distillation solution provides a customizable single pane view of both structured and unstructured data stores for over 950 file types with visualization and classification of all file properties. D3 crawls your entire data estate and uses artificial intelligence and machine learning to see risks hidden in actual file content – not just file attributes. D3 includes:
- Risk filters
- Data tagging and workflows
- Standard and user-defined metadata
- Federated search
- Alerts and automated monitoring
- Data Subject Access Requests (DSARs)
- Advanced queries – search for PHI/PII, or perform other sensitive or risky data searches for unencrypted intellectual property or exposed credentials
We invite you to test out data discovery on your own data with a free 1 TB Test Drive of Anacomp’s D3 AI/ML Data Discovery Solution.
Anacomp has served Fortune 500 companies and the U.S. government with data visibility, digital transformation, and OCR intelligent document processing projects for over 50 years